Subscribe to our newsletter
NLP Series: AI in Science; the Promise, the Challenge, and the Risk
Continuing our blog series on Natural Language Processing, Dr Joris van Rossum focuses on AI in science; the potential to make research better, but also the pitfalls that we must be wary of when creating and applying these new technologies. Joris has over 20 years of experience driving change in the publishing industry through new technologies and business models. His former roles include Director of Publishing Innovation at Elsevier and Director of Special Projects at Digital Science, a role in which he authored the Blockchain for Research report. He co-founded Peerwith in 2015, and currently serves as Research Data Director at STM, where he drives the adoption of sharing, linking and citing data in research publications.
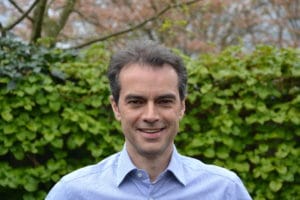
Understanding the risks
According to Professor Thomas Malone, Director of the MIT Center for Collective Intelligence, AI should essentially be about connecting people and computers so that they collectively act more intelligently than any individual person, group or computer has ever done before. This connectivity is at the core of science and research. Science is a collective activity par excellence, connecting millions of minds in space as well as time. For hundreds of years, scientists have been collaborating and discussing their ideas and results in academic journals. Computers are increasingly important for researchers: in conducting experiments, collecting and analyzing data and, of course, in scholarly communication. Reflecting on this, it is perhaps surprising that AI does not play a bigger role in science today. Although computers are indispensable for modern scientists, the application of artificial intelligence lags behind other industries, such as social media and online search. Despite its huge potential, uptake of AI has been relatively slow. This is in part due to the nascent state of AI, but also to do with cultural and technological features of the scientific ecosystem. We must be aware of these in order to assess the risks associated with unreflectively applying artificial intelligence in science and research.
AI and NLP in healthcare
A logical source of data for intelligent machines is the corpus of scientific information that has been written down in millions of articles and books. This is the realm of Natural Language Processing (NLP). By processing and analyzing this information, computers could come to insights and conclusions that no human could ever reach individually. Relationships between fields of research could be identified, proposed theories collaborated on or rejected based on an analysis of a broad corpus of information, and new answers to problems given.
This is what IBM’s Watson has attempted in the field of healthcare. Initiated in 2011, it aims to build a question-and-answer machine based on data derived from a wealth of written sources, helping physicians in clinical decisions. IBM has initiated several efforts to develop AI-powered medical technology, but many have struggled, and some have even failed spectacularly. What this lack of success shows is that it is still very hard for AI to make sense of complex medical texts. This will therefore most certainly also apply to other types of scientific and academic information. So far, no NLP technology has been able to match human beings in comprehension and insight.
Barriers to information
Another reason for the slow uptake of NLP in science is that scientific literature is still hard to access. The dominant subscription and copyright models make it impossible to access the entire corpus of scientific information published in journals and books by machines. One of the positive side effects of the move towards Open Access would be the access to information by AI engines, although a large challenge still lies in the immaturity of NLP to deal with complex information.
More data give greater context
Despite the wealth of information captured in text, it is important to realize that the observational and experimental scientific data that stands at the basis of articles and books is potentially much more powerful for machines. In most branches of science the amount of information collected has increased with dazzling speed. Think about the vast amount of data collected in fields like astronomy, physics and biology. This data would allow AI engines to fundamentally do much more than what is done today. In fact, the success of born-digital companies like Amazon and Google have had in applying AI is to a large extent due to the fact that they have a vast amount of data at their disposal. AI engines could create hypotheses on the genetic origin of diseases, or the causes for global warming, test these hypotheses by means of plowing through the vast amount of data that is produced on a daily basis, and so to arrive at better and more detailed explanations of the world.
Shifting the culture around data sharing to create better AI
A challenge here is that sharing data is not yet part of the narrative-based scholarly culture. Traditionally, information is shared and credit earned in the form of published articles and books, not in the underlying observational and experimental data.
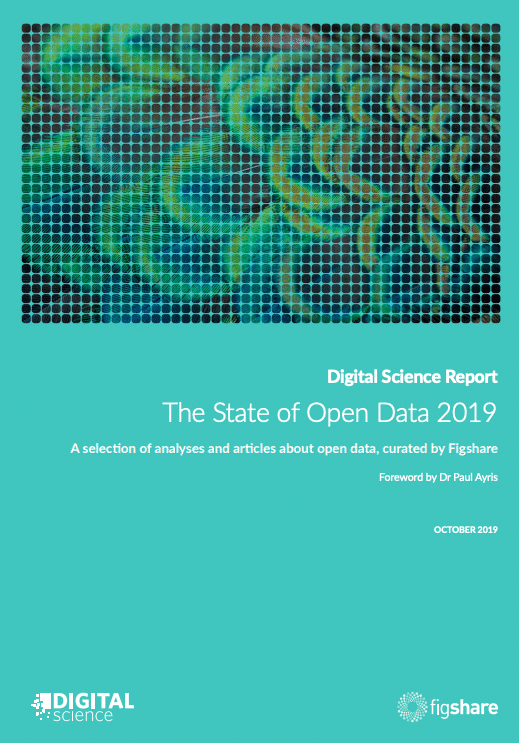
Important reasons for data not being made available is the fear of being scooped and the lack of incentives, as the latest State of Open Data report showed. Thankfully in recent years efforts have been made to stimulate or even mandate the sharing of research data. Although these offers are primarily driven by the need to make science more transparent and reproducible, enhancing the opportunity for AI engines to access this data is a promising and welcome side-effect.
Like the necessary advancement of NLP techniques, making research data structurally accessible and AI-ready will take years to come to fruition. In the meantime, AI is being applied in science and research in narrower domains, assisting scientists and publishers in specific steps in their workflows. AI can build better language editing tools, such as in the case of Writefull, who we will hear from in the next article in this series. Publishers can apply AI to perform technical checks, such as in Unsilo, scan submitted methods sections for assessing the reproducibility of research, the way Ripeta and SciScore do, and analyze citations, like Scite. Tools are being developed to scan images of submitted manuscripts to detect manipulation and duplication, and of course scientists benefit from generic AI applications such as search engines and speech and image recognition tools. Experiments have also been done with tools that help editors in making decisions to accept or reject papers. The chance of publishing a highly cited paper is predicted based on factors including the subject area, authorship and affiliation, and the use of language. This last application exposes an essential characteristic of machine learning that should make us cautious.
Breaking barriers, not reinforcing them
Roughly speaking, in machine learning, computers learn by means of identifying patterns in existing data. A program goes through vast numbers of texts to determine the predominant context in which words occur, and uses that knowledge to determine what words are likely to follow. In the case of the tools that support editors in their decision to accept or reject papers, it identifies factors that characterize successful papers, and makes predictions based on the occurrence of these factors in submitted papers. This logically implies that these patterns will be strengthened. If a word is frequently used in combination with another word, the engine subsequently suggesting this word to a user will lead to that word being used even more frequently. If an author was successful, or a particular theory or topic influential, AI will make these even more so. And if women or people from developing countries have historically published less than their male counterparts from Western countries, AI can keep them underperforming.
In other words, AI has the risk of consolidating the contemporary structures and paradigms. But as the philosopher of science Thomas Kuhn showed, real breakthroughs are characterized by replacing breaking patterns and replacing paradigms with new ones. Think of the heliocentric worldview of Kepler, Copernicus and Galileo, Darwin’s theory of natural selection, and Einstein’s theory of relativity. Real progress in science takes place by means of the novel, the unexpected, and sometimes even the unwelcome. Humans are conservative and biased enough. We have to make sure that machines don’t make us even more so.
DOI: https://doi.org/10.6084/m9.figshare.12092403.v1