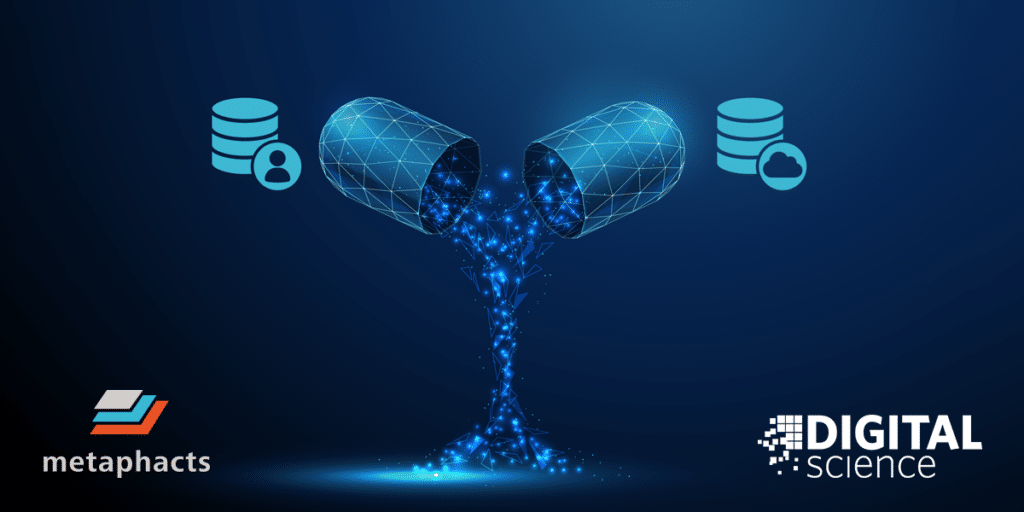
Despite the increasing availability of public data, why are so many pharma and life sciences organizations still grappling with a persistent knowledge divide? This discrepancy was a focal point at the recent BioTechX conference in October, Europe’s largest biotechnology congress that brings together researchers and leaders in pharma, academia and business. Attendees and presenters voiced the same concern: the need to connect data from different sources and all internal corporate data through one, integrated semantic data layer.
For example, combining global public research with proprietary data would provide pharmaceutical companies with valuable knowledge that could help drive significant advancements in research and product development. Linking to public knowledge can enrich existing internal research with metadata and contextual meaning, and equip decision-makers with new insights, context and perspectives they might not have had access to originally, leading to more strategic and informed decisions. For instance, it could help fast-track target discovery and reduce research costs or streamline processes from R&D to clinical trials to market access.
Additionally, this integration of data through a semantic layer unlocks the potential to drive many AI solutions across the pharma value chain and embed a layer of trustworthiness and explainability in these applications. Having reliable and precise AI solutions is critical, especially in the pharma sector, which deals with sensitive and high-stakes use cases, such as using AI to discover hidden relations between drugs, genes, diseases, etc., across multiple datasets, clinical trials or publications, for example.
Using fragmented data could cause AI to miss essential connections, or at worse lead to inaccurate predictions regarding drug interactions or outcomes.
Several root causes contributed to this gap between the public and private data spheres, including an absence of suitable infrastructure and technology to connect disparate data and global public research, and a lack of tools to contextualize retrieved data and derive meaning from it.
Integrating public knowledge
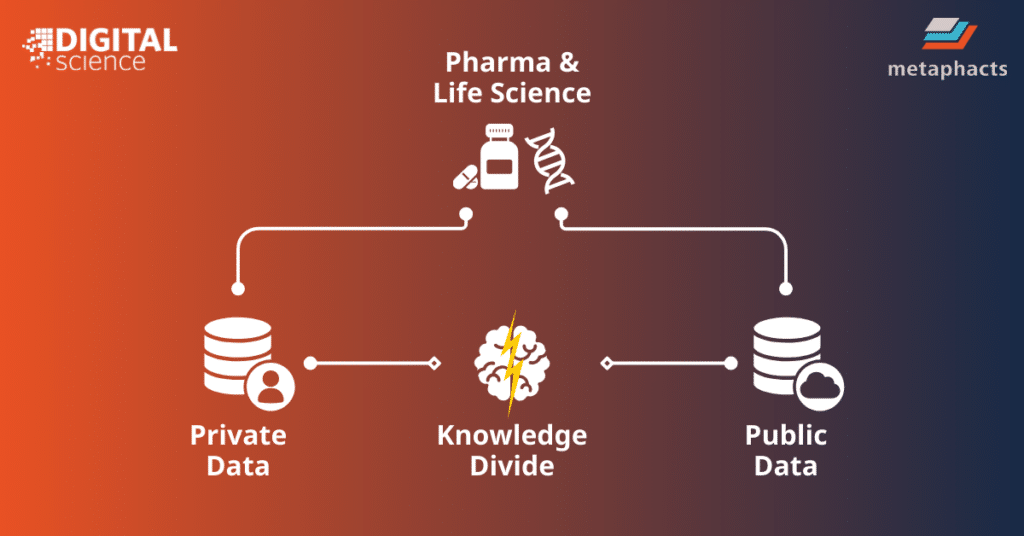
Although a considerable amount of research is public and open, many companies don’t have the necessary software or technology (though these are now widely available) to access these vast datasets, while for other research they require a license for full access to the available literature.
Even when full access is permitted, there is still a time and labor expenditure that impedes the immediate use of this data. For example, for companies that have dedicated internal resources to deep dive into scientific literature reviews, these literature research reports can take weeks or even months to complete because a (human) worker will need to review hundreds or thousands of these sources manually. Additionally, new data is being published constantly, making it difficult to keep up with emerging research.
Many pharma, biotechnology or medical devices organizations will also outsource research work to Contract Research Organizations (CROs) that carry out services such as clinical trials management or biopharmaceutical development, with the aims of simplifying drug development and entry into the market. However, collaborating with CROs can require extensive back-and-forth, from sharing data and results to constant meetings and email communication. As a result, the fragmentation between the company and CRO can lead to notable delays, miscommunication, or at worse, culminate in incorrect decision-making.
Fragmentation within public research
A divide exists not only between private and public data, but this fragmentation also occurs within public research, thus exacerbating the issue. For example, several datasets can stem from the same research. To establish connections between these datasets, they need to be extensively reviewed, cross-referenced and analyzed. However, many of these public datasets do not integrate well with each other or other public sources, such as KEGG (Kyoto Encyclopedia of Genes and Genomes) and GWAS (Genome-Wide Association Studies) Catalog, for various reasons, including a lack of a standardized format or insufficient annotation, for example. Consequently, linking the metadata from these sources becomes challenging, making it hard to gain a clear understanding of the relations between them.
The quality of public research can also vary. There’s a considerable amount of manually curated data available that doesn’t provide evidence (i.e. data from clinical trials) or fails to cite the original report/document from which it is referencing, making it challenging to validate the accuracy, quality and conclusions drawn from the data.
Internal data silos
When leveraging corporate data, companies in the pharma and life sciences space have individuals, teams and departments all producing valuable data that could be used immediately or in the future, such as in the later stage of the pharma life cycle. For example, data from clinical trials for one drug could be extremely valuable in understanding the application of a target (e.g. protein) with another disease (e.g. a side effect in one trial could become a targeted disease in another), this data can also be used for drug safety review or commercial purposes later on. However, this data becomes difficult to share and repurpose later on if it lacks the original meaning and context in which it was created (which it often does). It might be presented in a spreadsheet without a clear legend or instructions on how to use or interpret the data. Also, data can get stuck within internal systems that require software and specialized technical expertise to retrieve or is buried within documents and personal emails. Retrieving data is also a time-staking and laborious task leaving little room for actual analysis and application of insights.
Knowledge drain
Once data is retrieved and supplemented with information and meaning, insights can be derived from the data, which is what we consider ‘knowledge’. Knowledge is the penultimate step in the decision-making process, right before a final decision can be made, making it an essential asset to any company.
Unfortunately, knowledge often becomes lost. This phenomenon, where knowledge becomes trapped or irretrievable, is something we call the Bermuda Triangle of Knowledge Drain. It refers to knowledge that gets swallowed into a vortex, swirling away into oblivion. When knowledge is left stuck in the minds of domain experts (who are unable to pass on their expertise due to a leave or departure from the company), when it’s confined to physical documents, slideshows and emails, or becomes isolated within siloed systems and software, it creates the perfect storm for a knowledge drain to occur.
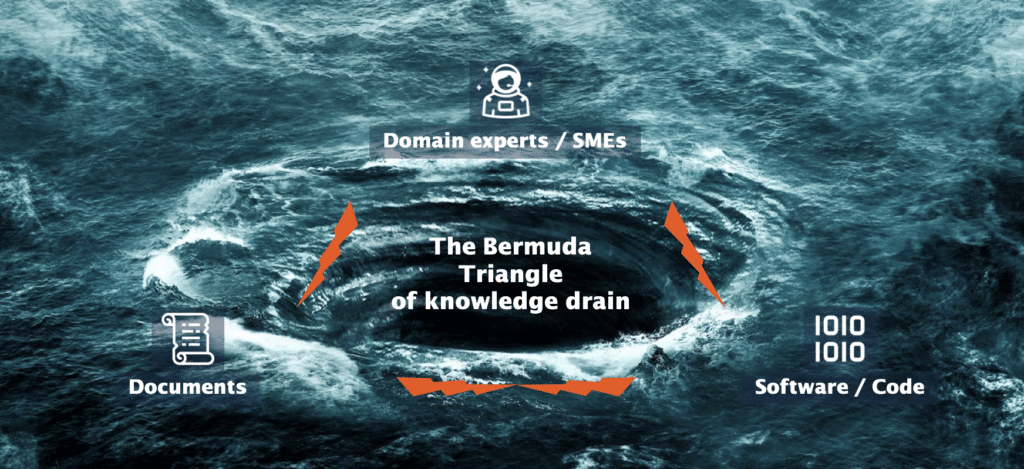
The solution is a knowledge graph. Knowledge graphs can bring together both private data and public research knowledge while addressing the challenges found within these two domains. It seamlessly connects to external datasets, utilizes existing metadata and ontologies, and imbues internal data with contextual meaning. The semantic layer within the knowledge graph allows you to connect to public data sources and transforms data into consumable, shareable and actionable knowledge while adhering to FAIR data practices, ensuring reusability and interoperability of data. As mentioned above, it also adds a trust and explainability foundation for AI applications, ensuring accuracy and eliminating potential hallucinations. This added layer of trust helps companies maximize existing AI investments and generate trustworthy and explainable AI solutions such as AI-driven drug discovery.
To conclude, the urgency and significance of addressing this fragmentation are unmistakable. Despite the existing challenges, the integration of public and private data presents substantial benefits for companies in the pharma and life sciences industries, which can be achieved through the implementation of a knowledge graph. A knowledge graph, such as the soon-to-be-launched Dimensions Knowledge Graph, can aid in streamlining the trial and manufacturing process, fast-tracking drug discovery, speeding up drug safety review processes and ensuring reusability of knowledge.
Stay tuned to learn about the Dimensions Knowledge Graph, a ready-made knowledge graph providing access to one of the world’s largest interconnected sets of semantically annotated knowledge databases while powering smart and reliable AI solutions across the pharma value chain.
For more information about knowledge graphs, check out this blog post by metaphacts.