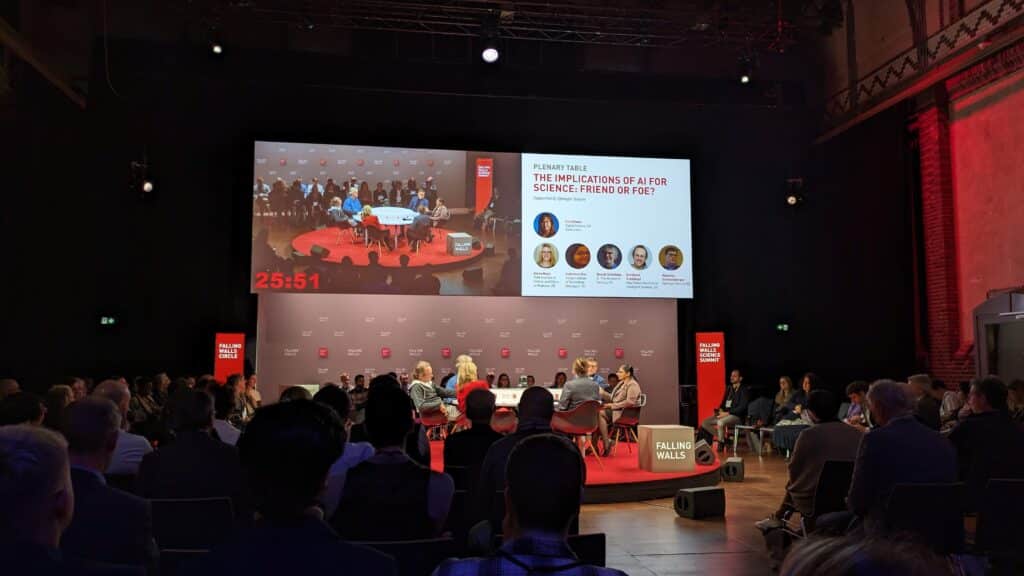
Update: Video recording of the session now available.
Well, Falling Walls certainly lived up to expectations! It’s six years since I was originally slated to attend but had to hand presentation duties over to my cofounder due to the birth of my youngest daughter, Annabelle.
I was fortunate to be able to attend in person this year, and today started with a wonderful panel session on the “Implications of AI for Science: Friend or Foe?”, chaired by Cat Allman who has recently joined Digital Science (yay!) and featuring a brilliant array of panellists:
- Alena Buyx, Professor of Ethics in Medicine and Health Technologies and Director of the Institute of History and Ethics in Medicine at Technical University of Munich. Alena is also active in the political and regulatory aspects of biomedical ethics; she has been a member of the German Ethics Council since 2016 and has been its chair since 2020.
- Sudeshna Das, a Postdoctoral Fellow at Emory University and with a PhD from the Centre of Excellence in Artificial Intelligence at the Indian Institute of Technology Kharagpur. Her doctoral research concentrated on AI-driven Gender Bias Identification in Textbooks.
- Benoit Schillings, X – The Moonshot Factory’s Chief Technology Officer, with over 30 years working in Silicon Valley holding senior technical roles at Yahoo, Nokia, Be.Inc and more. At X, Benoit oversees a portfolio of early-stage project teams that dream up, prototype and de-risk X’s next generation of moonshots.
- Henning Schoenenberger, Vice President Content Innovation at Springer Nature, who is leading their explorations of AI in scholarly publishing. He pioneered the first machine-generated research book published at Springer Nature.
- Bernhard Schölkopf, Director of the Max Planck Institute for Intelligent Systems since 2001. Winner of multiple awards for knowledge advancement, he has helped kickstart many educational initiatives, and in 2023 he founded the ELLIS Institute Tuebingen, where he acts as scientific director.
Cat Allman herself, now VP Open Source Research at Digital Science, was the perfect facilitator of the discussion – she has spent 10+ years with the Google Open Source Programs Office, and has been co-organizer of Science Foo Camp for 12+ years.
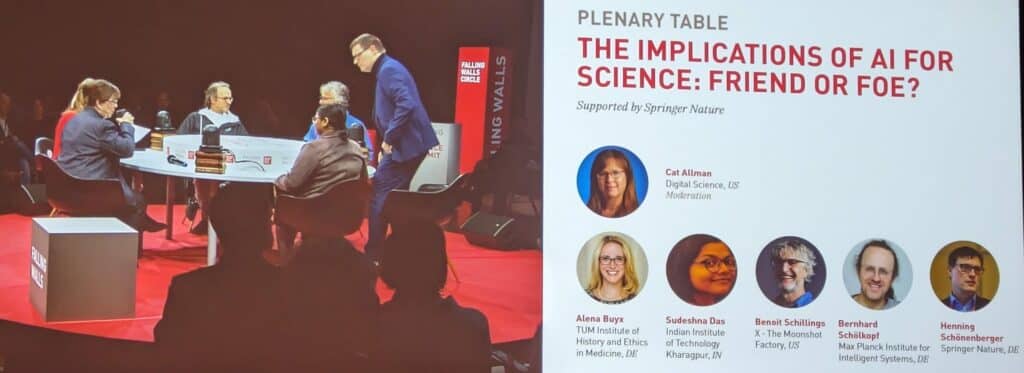
The panel session is part of Falling Walls Science Summit 2023, an annual event that gathers together inspirational people from across the globe who are helping to solve some of the world’s biggest problems through their research, new ventures, or work in their local community. I saw around 50 presentations yesterday during the Pitches day, and I’ll be sharing some of the highlights in a follow up post!
But before we go further, an important moment happened during the discussion, and Alena deserves a special mention for ensuring that Sudeshna was given the time to speak, just before the panel answered questions in the Q&A section.
Sudeshna had been unfortunately cut off due to timekeeping, and although it had been well-intentioned — to ensure the Q&A section didn’t get lost — Alena did the right thing in stepping in. Alena’s polite but firm interjection was appreciated by everyone in the room, and it’s this kind of thoughtfulness during the discussion, which was on show throughout, that made it a very enjoyable panel debate to attend.
Onto the session itself, and in their opening remarks, each panellist was encouraged to state whether they felt AI was a friend or foe to science. Of course, that is a binary way to view a complex and ever evolving topic, and the responses reflected this — they radiated a generally positive view of the potential for AI to help science, but with caution on how it’s important to focus on specific examples and situations, to try to be precise both in terms of what the AI is and what it’s intended to do.
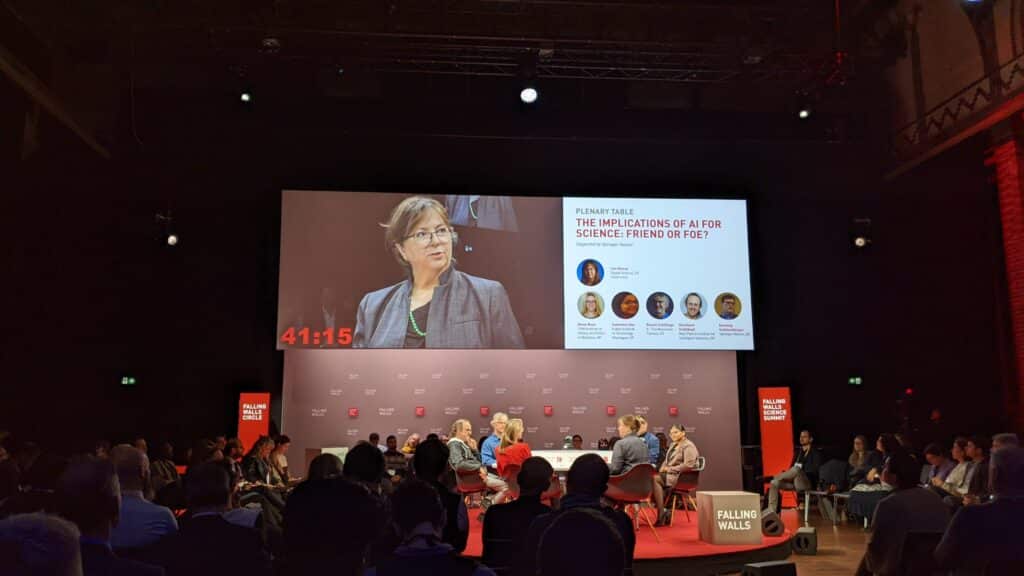
Benoit expanded on this need to be precise by giving a couple of specific examples of how he’s been experimenting with AI, both of which fall into the broader category of AI acting a personal assistant.
In one experiment, Benoit fed a model his reading list and asked for a personalised list of research recommendations and summaries. He was essentially taking to a more personalised level the types of recommendation engine that many websites use to (try to) encourage us to consume more content. What came across was his optimism that such a way of filtering / tailoring the literature — as an aid to a practising researcher — could help deal with the mountain of scientific content. He expects these types of systems to be common within the next few years, and it will be interesting to see who manages to create (or integrate) such a system successfully.
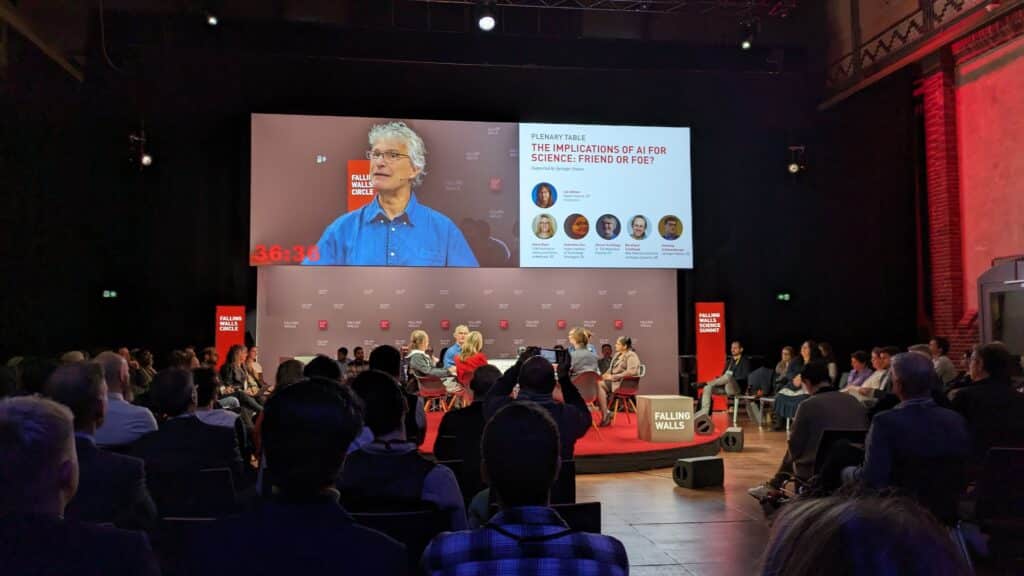
Whilst his first example could be seen as using an AI assistant to narrow down a broad selection of options, his second example is the reverse — when starting out on a new research topic, he often asks Bard for fifteen ideas for avenues to explore on that topic (I forget the exact phrase he used, sorry!). Although not all fifteen suggestions make sense, what comes back is usually useful at stimulating his further thought and ideas on the topic — it’s a great way to get started, and to avoid getting too deep or narrow too soon on a new project.
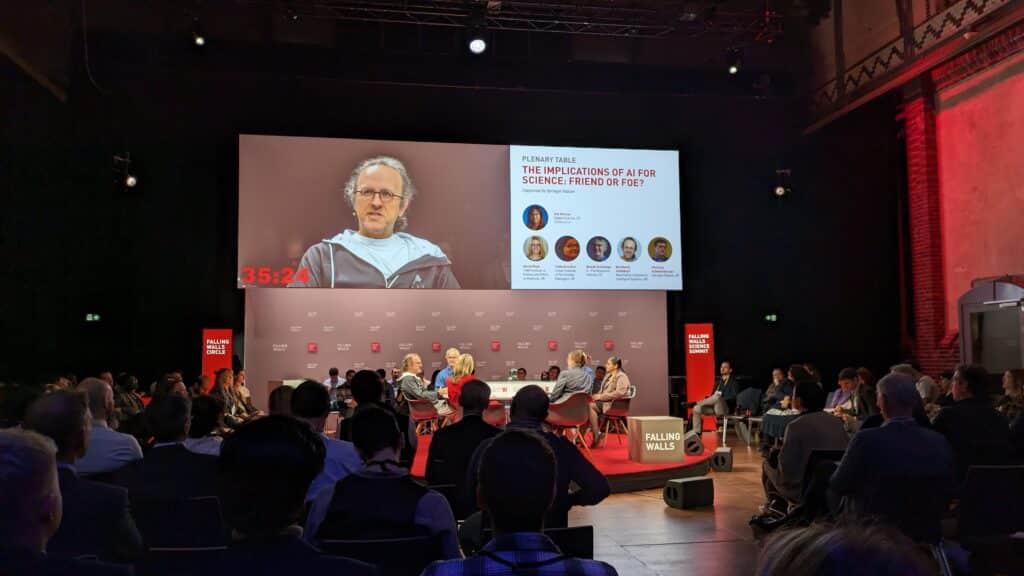
This issue with AI assistants giving incorrect or nonsensical answers also prompted the conversation to move onto that topic; Bernhard and his team are working on how future models could have some sense of causation, rather than just correlation, to try to help address this gap in current AI systems.
He gave a particular example where machine learning models had been trained to identify patients with a particular type of illness (I didn’t catch the name); when trained, the model appeared to give excellent detection rates, and appeared to be able to determine with high accuracy whether or not a given patient suffered from this illness.
However, when it was used in a clinical setting on new patients (presumably as a first test of the system), it failed — what had gone wrong? It turned out the model had spotted that patients with a thoracic (chest) tube had the illness, but those without the tube didn’t — as once a patient is being treated for the illness, they have such a tube fitted. As all the training data was based on known patients, it had used the presence of the tube to determine whether they had the illness. But of course new, unknown patients do not have a tube fitted, and hence the model failed. If models could have some sense of causation, this type of issue might be avoided.
This brings me onto one of the most interesting points raised during the discussion — Alena, who is a trained medical doctor, made the case that, rather than looking to AI assistants to help with potentially complex medical diagnoses, a real, tangible benefit to doctors all around the world would be for AI to help with all note-taking, paperwork, and admin tasks that eat up so much of a doctor’s time and energy.
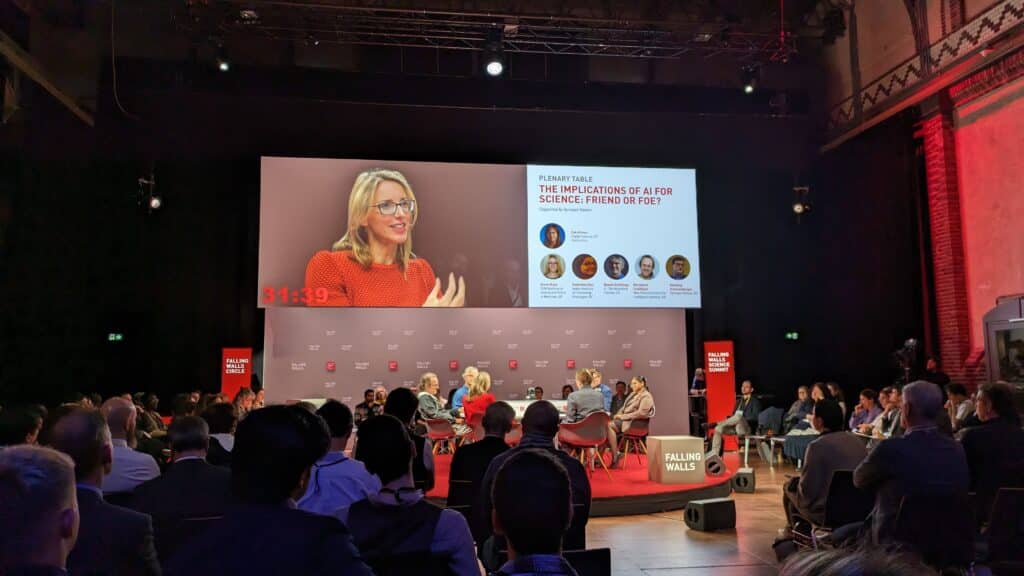
She made the point that there are other problems with having AI / automated diagnosis machines, namely that you end up with a layering of biases.
- First there is the algorithmic bias, from the machine learning model and its training data. For example, in medicine there are issues with training data not being gender balanced, or being dominated by lighter skin tones, making the results less reliable when applied to a general population.
- And secondly, there is the automation bias — that causes humans to trust the answer from a machine, even when it contradicts other observations — which adds a further bias on top. This combination of biases is not good for doctors, and not good for patients!
As an aside: there was a discussion on how the term “bias” is now often used almost exclusively to refer to algorithmic bias, but there is also inductive bias, which perhaps needs a new name!
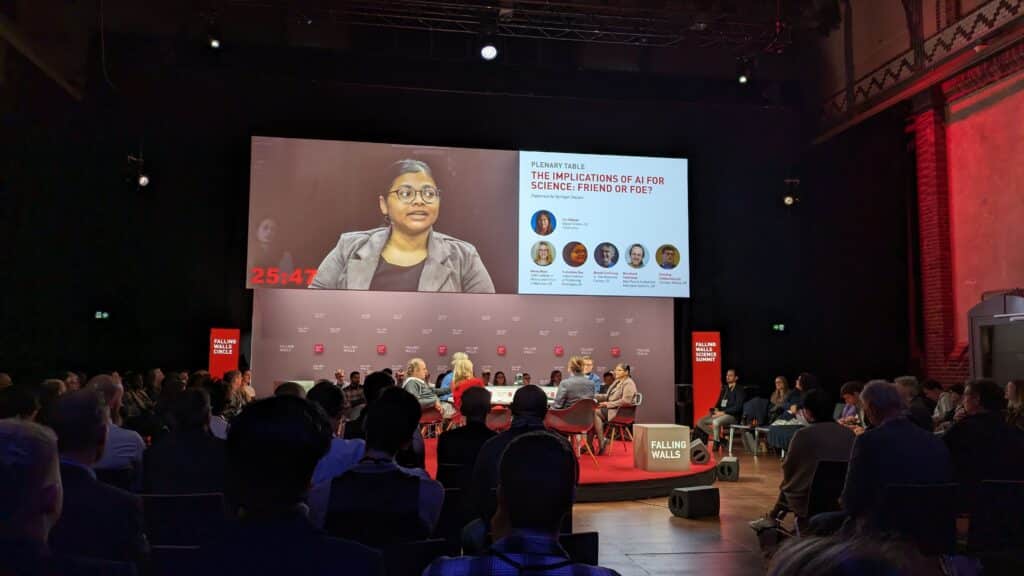
Sudeshna, whose PhD was in identifying gender bias in textbooks, was asked to comment on the issue of biases in AIs. She emphasised that results from AI models reflect biases present in the training data which generally reflect biases in human society. These biases can be cultural and/or driven by data-quality (garbage in -> garbage out), but also stem from the data tending to be from the Global North, where they lack local data from the rest of the world.
Henning gave an example where his team had seen a similar issue when testing a model on answering questions about SDGs; the answers were extracted from the literature which is predominantly focused on SDGs from a Global North perspective. Henning and I were speaking to Carl Smith in the hallway after the talk, and Carl mentioned how in psychology research this type of issue is often termed the WEIRD bias; another term I learned today!
Having local data — at different scales — is important for AI models to generate answers in context, and without that data, it’s hard to see how local nuance and variety won’t be lost. However, there’s no simple solution to this, and whilst a comment was made that improving data quality (labelling, accuracy, etc) — and training models based on high quality data — was one of the best routes to improving performance, it was acknowledged that it can’t by itself fix the issues of datasets only representing a small fraction of the world’s population.
Overall the tone of the discussion was one of cautious optimism though, and the examples given by the panellists were generally positive instances of people using this new technology to help humans do things better, or quicker, or both.
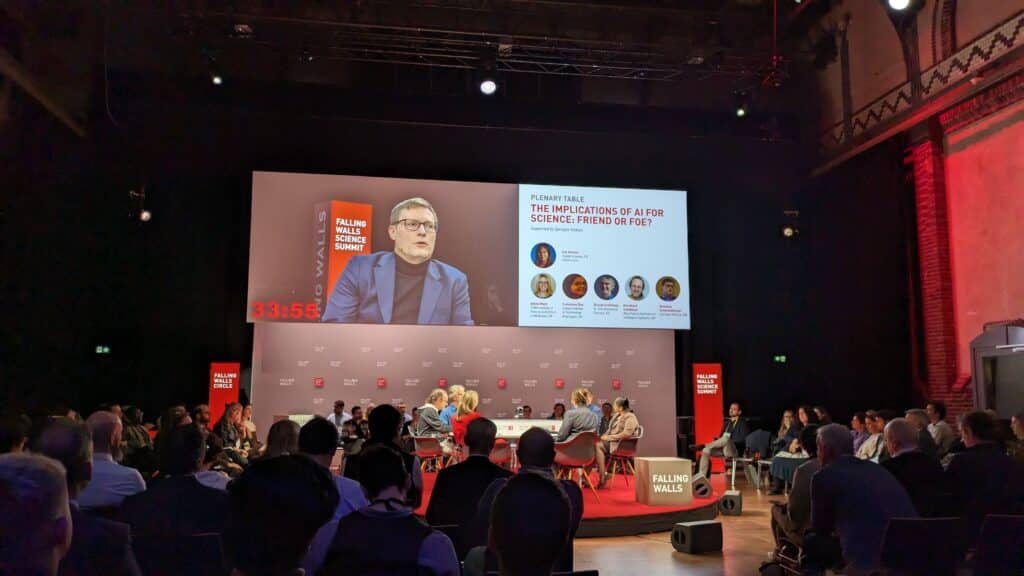
Earlier in the session, Henning had referred to a book recently published by Springer which was generated using GPT, but which crucially had three human authors/editors who took responsibility (and accountability) for the published work.
“This book was written by three experts who, with the support of GPT, provide a comprehensive insight into the possible uses of generative AI, such as GPT, in corporate finance.”
Translated opening sentence from the book’s description
Henning made a point of highlighting how current responsible uses of AI all have “humans-in-the-loop”, emphasising that AI is helping people produce things that they might not have the time or resource to. In this specific example, the book was written in approximately two to three months, and published within five — much shorter than the usual twelve months or more that a book usually takes.
There was time towards the end for a small number of audience questions, and the first was whether we had (or could) learn something from the previous time new technology was unleashed on the public via the internet and had a transformative effect on the world; namely the rise of social media and user generated content and interaction, often dubbed Web 2.0.
It was at this point that Alena stepped in and gave Sudeshna the time to add her thoughts on the previous topic, that of how to address bias in the large language models.
Sudeshna made the very important comment that there is no fixed way in how we should look to address biases, because they aren’t static; the biases and issues we are addressing today are different from the ones of five or ten or twenty years ago. She mentioned her PhD study, on gender bias, and how today she would take a broader approach to gender classification. And so whatever methods we determine for addressing bias should reflect the fact that in ten years we will very likely see different biases, or see biases through a different lens.
Alena then gave a brilliant response to the question of whether anything was different this time vs when Facebook et al ushered in Web 2.0.
She said that back then, we’d had the unbridled optimism to say “go ahead, do brilliant things, society will benefit” to those tech companies. Whereas today, whilst we still want to say “go ahead, do brilliant things” to those companies, the difference is that today we – society / government / the people — are in the room, and have a voice. And that hopefully, because of that, we will do things better.
As the panel wrapped up, Bernhard made the observation that early predictions of the internet didn’t necessarily focus on the social side, and didn’t predict how social media would dominate. He suggests we view our predictions on AI in a similar way; they are likely to be wrong, and we need to keep an open mind.
Finally, Henning closed out the session with a reminder that it is possible to take practical steps, at first an individual then organisational level, which set the approach across a whole industry. His example was that of the SpringerNature policy of not recognizing ChatGPT as an author, which came about because they saw ChatGPT start to be listed as an author on some papers, and very quickly concluded that, because ChatGPT has no accountability, it cannot be an author. Other publishers followed suit, and the policy was effectively adopted across the industry.
It makes you wonder what other steps could we take as individuals and organisations to bring about the responsible use of AI we all hope to encourage.
Disclaimer: The write up above is based on my recollection of the panel discussion and some very basic notes I jotted down immediately afterwards! I have focused on those points that stood out to be, and it’s not meant to be an exhaustive summary of what was discussed. I have also probably grouped things together that were separate points, and may have things slightly out of order. But I’ve strived to capture the essence, spirit and context of what was said, as best I can — please do ping me if you were there and think I’ve missed something!
Double disclaimer: For completeness I should point out that — as you can probably tell — I work at Digital Science, alongside Cat. Digital Science is part of the Holtzbrinck Group, as is Springer Nature, who supported the session. But the above is entirely my own work, faults and all.