For the last 15 years the scholarly communications industry has been trying to deal with the problem of predatory journals. Could the advent of Generative AI provide a solution? Publication ethics expert Simon Linacre shares the good and the bad news on this question, and reveals it may not be just a matter of trust in academic publishing, but knowing where to find it.
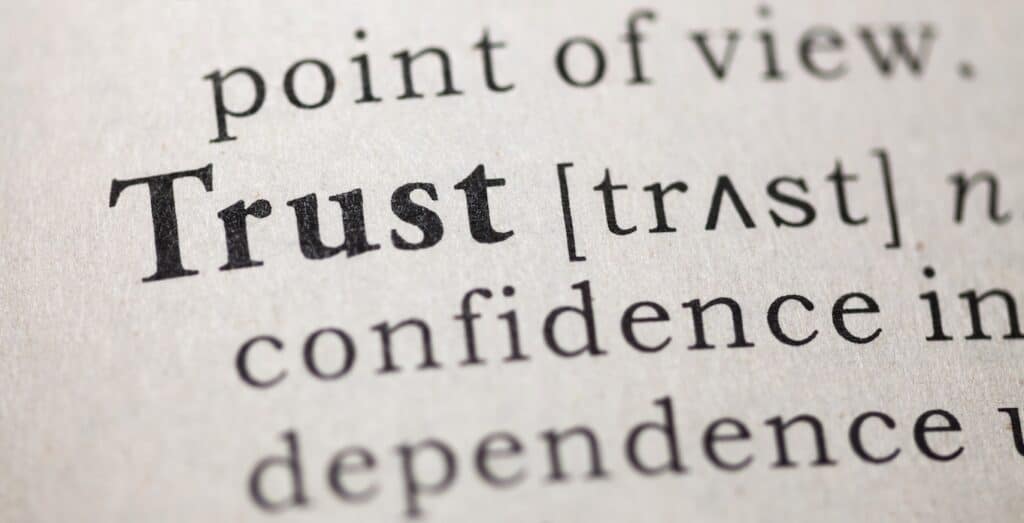
Predatory journals can be identified as deceptive and often fake, giving the appearance of legitimate peer-reviewed journals. In researching my book The Predator Effect on the subject, I found the whole practice irredeemably rotten, and the very antipathy to the notion of trust that glues together the research ecosystem on which we depend. A predatory journal is what happens when every element of trust is corrupted in the pursuit of knowledge: research is not done thoroughly; authors are deceived; peer review is ignored; outcomes reduced to box-ticking exercises.
The existence of predatory publishers was first discussed 15 years ago in 2008 by Gunther Eysenbach in the article ‘Black Sheep Among Open Access Journals and Publishers’, and Jeffrey Beall first coined the term ‘predatory journal’ in 2010. The use of language such as ‘predator’ and ‘black sheep’ has perhaps added a hint of intrigue around the activity, yet predatory publishers are not modern day pirates or Robin Hoods. It was estimated in 2020 that over $100m a year was being lost to predatory publishers, much of it from funders. And that’s not to mention wasted publications of good research that are stigmatized in predatory journals, or questionable research that has been cited but not validated by peer review.
Is AI the answer?
Two recent pieces published by Digital Science CEO Daniel Hook have addressed the need for skilled prompt engineers to develop Generative AI solutions successfully, and how early AI Art is having issues with some seemingly simple instructions and bias. From these, we can see that AI still has a way to go to engender our full trust, nevertheless it has produced some transformative illustrations of its potential value. But could it prove an antidote to the predatory publishing problem, and thereby increase trust in the publishing process?
When we think about the predatory journal issue, given the ability of Large Language Models (LLMs) to generate convincing text at zero cost to the user, this threatens the business model of the deceptive publisher. There are only a few studies into the motivations of authors who publish in predatory journals, but those that have looked at the question broadly identify them as either being unaware or unethical. While a naïve author may still publish in a predatory journal thinking it is legitimate, an unethical one may weigh up the expense and risk of knowingly doing so against the cheaper and potentially less risky alternative of using Generative AI.
For example, imagine you are an unethical author and just want to get a publication in a recognized journal, and are willing to take some risks to do so, but unwilling to actually write a real paper yourself. Publishing in a predatory journal is an option, but it will cost a few hundred dollars and only gives you a publication in a journal few people have heard of, so significant risk for minimal reward. However, if you ask an AI to write you a paper and then make some tweaks, you might get it published in a better journal for no fee (assuming you take the non-open access route). With AI-checking software still in its infancy, an unethical author may decide that using an AI is the most effective course of action, hoping it escapes detection and is accepted. And of course, they can much more easily increase their output using this option. So as we can see, an unintended consequence of Generative AI could be to reduce demand for predatory journals, effectively disrupting their business models.
Trust still the victim
While this mitigates the success of bad actors in the publishing field, however, it may only do so at the risk of further impacting the already growing problem of plagiarism and paper mills in mainstream academic publishing. Recent large-scale retractions have revealed an iceberg of ethical concerns as a result of paper mills, with many more problems being revealed as investigations become better equipped at spotting fake publications. But this is all after the fact. For the sake of science and scientific communications, we need to increasingly look for trust, and not rely on old faiths where trust was taken for granted.
So, what might trust look like in a post-AI world? One example would be the new Dimensions Research Integrity solution, which enables you to identify Trust Markers across a researcher’s or an organization’s academic outputs. Trust Markers are verifiable elements recorded in a publication that represent the transparency and reproducibility of scientific research. As new technology such as LLMs and Generative AI grow in influence, previous bad actors such as predatory publishers may be diminished. However, new challenges to trust and research integrity in scholarly communications will replace them, and knowing what trust in research is and where to find it will only become even more important.
Dimensions Research Integrity